The geo-enabled Internet of Things can make city-planning and decision-making more efficient, says Ravi Nishesh Srivastava
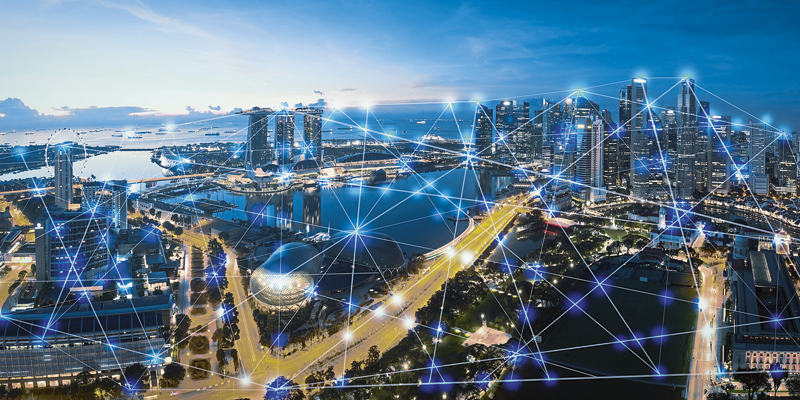
The Smart City concept is a global vision for the development of a city’s infrastructure and its management and use. It is a gateway between the government and the citizens that undergo several reforms and a retrofit process on existing city scenarios.
Cities across the globe are going through the reforms to meet the Smart City criteria that require a four-sided technological foundation and support. It is a fact that a single technology or platform cannot serve all a city’s needs. Distributed systems for cities’ requirements need additional effort, cost, resources and an elaborate maintenance plan, which typically result in inefficiencies in usage.
GIS has been a strategic tool for city authorities to develop quick and efficient processes for planning and decision-making. Most strategic initiatives involved GIS in their workflows for easy decisions through visualisation. From an era of hard-copy maps to an age of digital online maps on smart devices, including 3D maps now, GIS has evolved by leaps and bounds in the technology space.
GIS has always been compatible with other technologies and domains. As far as sectors are concerned, GIS has no limitations, ranging from oil and gas, the environment and natural resources to utilities, defence and forestry. From time to time, GIS enhances itself from a technological perspective and aligns with existing needs, and the Internet of Things (IoT) has been sending out strong signals across the globe for reforming cities through the power of connected devices and the Internet.
Thus, we have G-IoT – the geo-enabled Internet of Things – a framework that joins 3D maps with the power of IoT to deliver a seamless information system for city authorities. A vital aspect of the G-IoT framework is to have 3D geospatial information, which provides a realistic perception of the city. The intelligence provided by the 3D data creates an accurate visual perspective, while its combination with IoT delivers a real-time view of the city.
3D GIS helps plan device location and deployment, as well as CCTV camera placements, thus enabling smart components in the city and their correlation with adjacent features. It also helps visualise the network reach, which looks accurate in a 3D map. Among the Smart City components mapped with IoT are noise pollution, temperature, rainfall, gas emission, solid waste and parking – almost everything that can transmit data.
The G-IoT framework therefore enables a user to map IoT devices in the field in a 3D manner, connect with the cloud, record their live feeds, set the rules for alerts and alarms, and provide valuable insights through dashboards.
Use case – environmental monitoring
Rapid urbanisation and industrialisation create environmental challenges concerning air quality, water, solid waste and more that need to be continuously monitored, and preliminary actions need to be taken by the city administration to build resilient cities.
The G-IoT framework empowers users from multiple departments to easily integrate, assess, analyse the information, and make quick and accurate decisions on city issues and requirements. This digitally transforms the complete environmental monitoring aspect and aligns it with the current technology trend. The digitalisation of resources such as infrastructure, devices, sensors, and networks further enables optimum usability and efficiency.
To accelerate the research and development of this framework, various factors need to be in place. These include:
- Various sources of geospatial data, including maps, attributes, relationship classes and 3D building models.
- Information on data requirements depending on the exact problem of air quality – indoor or outdoor. There are specific data formats for various content standards and models, such as IndoorGML (for indoor 3D data) or CityGML.
- Sensor datasets transmitting through the network and mapped to locations through a data stream.
- A well-defined algorithm to describe use case functions.
Using this framework, indoor air quality monitoring, a critical use case, can be adequately addressed and used to monitor spaces, occupancy, the air quality index (AQI) and other factors. IndoorGML is an open standard for an open-data model and
XML schema for indoor-spatial information. It aims to provide a common framework of representation and exchange of indoor spatial information. This representation deals with the interior of buildings from geometric, cartographic, and semantic viewpoints. IndoorGML deliberately focuses on modelling indoor spaces for navigation and air quality measurement purposes.
Location perspective
Visualisation is not the only requirement in the 3D space. Several real-time use cases also justify the 3D experience – smart environment, smart mobility, smart governance and smart communications combine with 3D models to provide a realistic outlook of any city.
A network of devices or sensors is not worth visualising unless it is linked to a location. Therefore, location perspective adds a real-world sense of its existence and enforces better planning and efficiency. A 3D representation is a value-add to the location, which helps to visualise the IoT components in their precise location and define their existence, height, direction and orientation. This enables better planning, outreach, management, maintenance and operations.
Visualisation
Any G-IoT-enabled system needs a proper visualisation platform to stream geospatial data and IoT data streams that also allow a dashboard to monitor the data and take appropriate actions. The visualisation engine is an essential part of this entire framework and is the front-end interface for city officials and users. The visualisation engine, when incorporated with various tools such as predictive analysis, temporal summary, integration endpoints for external systems such as CCTV feeds and other data inventories, make this entire framework more effective for cities.
Device health monitoring is another vital aspect that has a direct impact on visualisation. Algorithms based on data continuity from the sensors can help share an alert with city officials enabling a quicker response time. The integration algorithm needs to collect latitude, longitude, and altitude details from the sensors and should contain the exact location on the map.
Conclusion
From enhancing operational efficiencies for the city administration and addressing environmental issues with insights and recommendations on AQI to providing visualisation for informed and effective decision-making, the world is now moving into a different dimension of visualisation. We have already seen and explored 2D maps with real-world use cases and we are now successfully incorporating 3D and 4D elements into the map for more efficient operations.
The example of conjoining the environmental monitoring aspect with GIS maps in real-time illustrates the capability of efficiently addressing and managing a vital aspect of the city. Seamless data and communication networks are the main pillars of such implementations, which can, in the future, move the entire concept to 7D.
Ravi Nishesh Srivastava is solution architect at Cyient (www.cyient.com)