A mass of infrastructure is hidden under our cities, much of it poorly mapped, if at all. Fraunhofer IPM is developing an AI-based system that recognises typical underground objects in image data. Alexander Reiterer reports on the progress so far
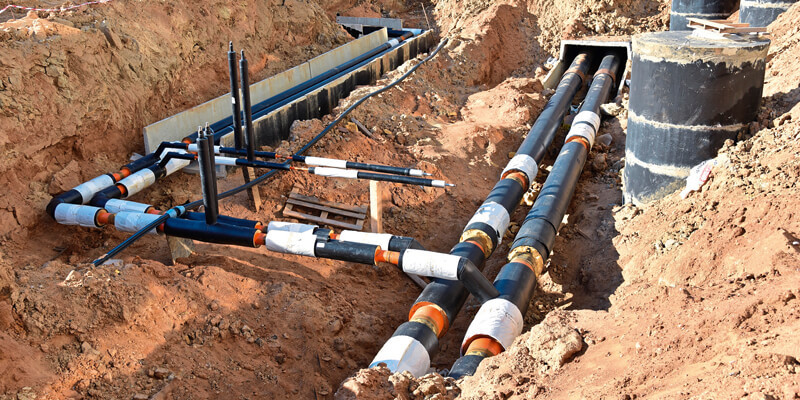
Gas pipelines, electric wires, communication cables, speed pipes for the fibre optic network, district heating lines – a whole maze of vital infrastructure is buried beneath our cities and landscapes. To ensure reliable planning and to prevent accidents, network operators are obliged to draw up precise site plans when laying lines.
While numerous solutions for automated mapping of above-ground infrastructure have become available over the past years, mapping of earthbound infrastructure is still done manually in the vast majority of cases. For small excavations, such as house connections, hand-drawn sketches are produced on site; larger excavations are measured using total stations or GNSS. Digitisation is managed later on by importing the drawings or the measurement files into a GIS. Post-processing the data in this way is not only time-consuming and inefficient; it also turns out to be unreliable, as sketches drawn by hand can never be as precise as data acquired by appropriate technology.
Partly automated imaging solutions have been emerging onto the market for some time now. However, they are mostly 2D and georeferencing has to be realised in an extra post-processing step by using known points, such as house edges or special ground-control points.
Fraunhofer IPM’s aim is to develop a fully automated, georeferenced recognition and quantification process for the documentation of underground supply networks. In its low-cost version, users of the technology can record a scene by using a handheld mobile device while walking along the construction site. For larger excavations, a UAV-based system records the scene from the air. Both approaches have to generate high-resolution data with as few gaps as possible. This data is used for recognition and reconstruction.
In addition to high demands on information density, dealing with difficult external conditions is also challenging. For example, camera systems reach their limits when the lighting situation is characterised by high dynamics, such as the drop shadow of a pit. We use multiple exposures and a fusion of 2D and 3D data to produce data of the highest quality. The recorded data is directly georeferenced, automatically interpreted and integrated into a GIS.
Efficient digitisation and 3D reconstruction
The construction site digitisation module consists of either a camera system or a laser scanning system. The workflow is implemented as a modular process with the possibility of using a wide range of different sensing systems. However, there are a few preconditions regarding the sensors. For example, the cameras have to be equipped with global shutters in order to compensate motion blur and differing exposure times due to changing lighting conditions. The software features a correction mode that gives feedback to the user as soon as the device is no longer correctly positioned to fully record the excavation pit.
The digitisation module comprises the software for 3D-reconstruction of the excavation pit based on the data stream. Algorithms have been developed that enable the reconstruction to be performed in real-time, while economically using the limited resources of the devices. This way, the user can directly assess the quality of the acquired data using a graphical user interface. In a subsequent process, the data stream is processed, resulting in a dense point-cloud with a great wealth of detail. The volume of the excavation pit can be directly calculated from this data. The data stream is stored for quality control and subsequent automated semantic interpretation, first on the device and later on in a data space centre. The system complies with all regulations regarding privacy protection when using cameras in public scenarios. Image data can be pixelated in real-time if required.
Georeferencing with precision in the centimetre range
A dynamic georeferencing module automatically annotates the recorded data with highly precise geolocation information. The DGM consists of a low-priced inertial measurement unit (IMU) for orientation and a GNSS system for determining the geo-position and is directly linked to the CSD. Special filter algorithms for the IMU data provide a topographically correct representation of the resulting 3D-pointcloud. Multiband GNSS receivers using GPS, GLONASS and Galileo are logged, allowing live georeferencing based on the raw data. Positioning accuracies of around 10cm are possible. A direct internet connection enables real-time correction of the GNSS data to achieve accuracies within a range of 5cm. If connection to the internet is unavailable, satellite meta-data is used to increase accuracy. A software add-on enables the system to use these correction services as soon as the device can re-establish a telecommunication connection. Furthermore, ground-control-points can be used during the measurement process. All measures combined, position accuracies of up to 1cm can be achieved.
Artificial intelligence
An object recognition software analyses the output data to classify the infrastructure elements. We are currently working on a deep learning algorithm to train an artificial neural network (ANN) for this special recognition task. A first prototype has already been developed. Instead of using commercially available databases such as Google AI, Microsoft Azure or IBM Watson, we are building up an extensive proprietary image database of cables and connectors to train the ANN. This database is being continuously extended by further data from field tests. An outdoor laboratory has been set up where the system is continuously tested and additional data for training the ANN is acquired. In the long run, data acquired by industry partners during surveying campaigns can also be used to continuously improve the machine learning process.
The object recognition software automatically classifies the different types of lines, tubes, cables or connectors, and quantifies them in terms of length and quantity respectively. The output of the neural network is stored and merged with the positioning information and meta-information on their exact type. Object recognition focuses on a set of defined object types and achieves a reliability of over 90% so far. The software can be trained for a broader variety of objects, such as various underground infrastructure elements used in countries other than Germany. In the long-term, we are aiming for a recognition rate of close to 100%. The system will provide a user interaction to change falsely detected infrastructure elements or add new elements to continuously improve and extend the system.
The automated process means a significant gain in efficiency compared to manual solutions: the time needed for surveying, interpreting the data and storing it in a GIS is reduced by around 25%.
The system will be used productively for the first customers in 2021 and will then be expanded. The expansion to further object types and the implementation of a proprietary human-machine interface is planned, so that new objects can be trained by customers without our support.
Alexander Reiterer is head of department, Object and Shape Detection, at the Fraunhofer Institute for Physical Measurement Techniques IPM (www.ipm.fraunhofer.de)