We are about to be swamped in location data. Analysing it will beyond human capability, so the OGC is examining how machine learning can be used instead. Ryan Ahola reports on the results so far and what tests are now being planned
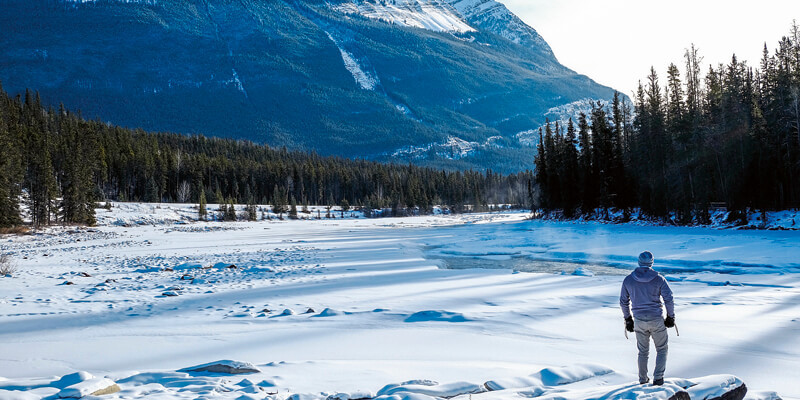
We are at the beginning of a revolution. Technologies such as UAVs, nanosatellites, earth observation satellites, 5G and the Internet of Things are set to become ubiquitous. That means location data will become ever more important in our lives, which poses a challenge for today’s mapping experts: how can this revolution improve existing and support new, mapping applications, and how can existing technologies support this fundamental change?
The volume of location data available today is massive. But future location-centric technologies such as 5G sensors and autonomous vehicles mean the world will experience exponential increases in location data.
Making sense of such vast quantities will not be possible with traditional, human-centric mapping approaches. Machine learning technologies will be critical to ensure we can continue to derive important insights from location data. To prepare for this future, the OGC has created a new working group and is fostering innovation through the OGC Testbeds.
OGC has created a community forum on artificial intelligence in geoinformatics. The OGC GeoAI Domain Working Group (GeoAI DWG) brings together geoscientists, computer scientists, engineers, entrepreneurs, and decision-makers from academia, industry and government to develop, share and research the latest trends, successes, challenges and opportunities in the field of AI and machine learning with geospatial data. The GeoAI DWG works interactively with the OGC Innovation Program, including making recommendations regarding innovation in OGC Testbeds.
To explore the potential of OGC standards to enable machine learning mapping, Natural Resources Canada has supported research in OGC’s Testbed-14 and Testbed-15 initiatives. These provide an agile, collaborative experimentation environment where the future of geospatial standards application and use can be explored.
OGC Testbed-14 provided the starting point for this effort with an examination of how machine learning can be supported with OGC web services, and how machine learning inputs and outputs can be published through OGC web services. The results suggested multiple recommendations for how OGC web services can better support machine learning, such as developing experimental web services to enable interoperability at the model level and improving web service interoperability with Earth observation exploitation platforms.
In OGC Testbed-15, the potential of OGC standards to enable machine learning applications for operational natural esources activities was explored. While a range of topics were examined, I will discuss two applications in detail.
Forestry management
Forestry operations are complex. Planning harvest activities requires consideration of many factors such as access (road availability), logistics, and weather (appropriate weather conditions for harvest and construction). With historical information and current trends available to provide insight into these considerations, there is an opportunity for machine learning to provide strategic information to augment the forestry planning process.
In OGC Testbed-15, a simplified experiment was proposed to determine if machine learning, supported by OGC standards, could optimise the delivery of forestry products from source to market. This encompassed product routing, road construction, and economic factors (historical lumber and fuel prices). Reinforcement lLearning was used due to the complexity of variables and the desired outcomes. A potential five-year spatial harvest plan scenario was developed through this approach.
Results of the forestry management experiment reveal that information provided through services implementing OGC standards can successfully enable a machine learning approach to provisionally plan forestry operations. While this work provided interesting ideas, additional research is necessary to determine how machine learning outcomes can be implemented within forestry operations to achieve practical results.
With a view towards this work, several improvements to OGC standards were proposed, including better support of dynamic, high frequency access to OGC Web Services, and advancing the implementation of OGC APIs to better support machine learning applications.
Web service discovery
Many organisations offer spatial information through web services that can be freely accessed. For example, approximately 3,500 web services representing about 86,000 layers, are provided by Canadian organisations and collated on the nrcan.gc.ca website.
Given this quantity and variety of data, discovering required information through factors such as theme or geographic location is important. Machine learning presents an opportunity to identify web services meeting specific criteria without having knowledge of their existence.
OGC Testbed-15 explored machine learning of available web services through geographic location. The aim was to identify services that provide spatial information for the Arctic. The machine learning approach used positive and negative training data to enable model recognition of typical characteristics of data representing Arctic and non-Arctic areas. Using a scoring approach, web services that fit the characteristics of Arctic data would be kept, with others rejected.
Application of the approach showed that web services meeting the Arctic geographic definition could be discovered without prior knowledge of their existence. While promising, substantial challenges were identified, particularly around the completeness of web service metadata. The presence of incomplete or inaccurate metadata resulted in improper positive identification by the model. This represents a challenging problem as machine learning approaches are only as good as the information they are provided. Ensuring web services can properly enable machine learning approaches will gain greater importance over time as increasing quantities of spatial information require automated approaches for access and use.
The future
Research has shown that OGC standards can support the use of machine learning for mapping – an important finding, as increasing data volumes make automated discovery and analysis approaches necessary. To advance the use of machine learning towards operational spatial analysis, Natural Resources Canada plans to continue its collaboration with OGC.
Our next endeavour is OGC Testbed-16 where the potential of OGC standards to support wildfire planning and response will be explored. With climate change increasing the frequency of devastating wildfires that impact many nations, understanding the potential of new technologies to help us manage these events is crucial. As geospatial standards will play an important role in the provision of information to manage these events, working to understand how they can enable new approaches is important for mapping experts and agencies to consider as they plan future activities.
Ryan Ahola is an environmental scientist at Natural Resources Canada. The author would like to thank Brian Low at the Canadian Forest Service for his help in putting this article together