Terri Freemantle and Tom Jones reflect on the rapid evolution of Analysis Ready Data and its ramifications for satellite-based Earth Observation
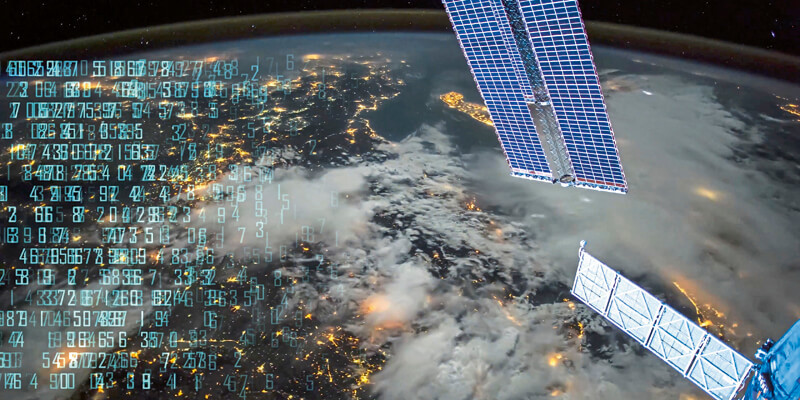
Within the last five years, the term ‘Analysis Ready Data’ (ARD) has become commonplace. The associated technologies have matured technically and conceptually both within the downstream Earth Observation (EO) community, as well as – perhaps more importantly – in other broader technology sectors, facilitating greater uptake and application of Earth Observation data sources for a wide range of use cases.
While translating the concept of ARD into practice is creating challenges and opportunities for both providers and users of geospatial image assets, a refined understanding of the evolution of the topic has become critical for organisations to offer viable value propositions across both the public, and private sector.
We can consider ARD as comprising three key components: (1) data formatting and hosting, (2) queryable metadata, and (3) scientific quality and provenance.
The ambition of organisations embracing the concept of ARD, as per the Committee on Earth Observing Satellites (CEOS), is to allow “immediate analysis with a minimum of additional user effort and interoperability both through time and with other datasets”1.
Data Formatting and Hosting
Regarding formatting and hosting, we’ve seen our community quickly, and openly, develop cloud-optimised data formats for use as standard across our toolsets. Cloud-Optimised GeoTIFF (COG)2, one such format, has recently been formalised as a GDAL driver. COGs can be seen in action at cogeo.org/map/.
COGs offer a very simple requirement for public and private data providers to expose datasets for maximum use within the web-economy: host datasets in a cloud-optimised format, leveraging object storage, accessible via a web interface.
Do traditional OGC protocols not address these requirements? The current answer is yes, and no. COG functionality enables client applications to efficiently request portions of an image – rather than download the entire file client-side. OGC web services offer the ability to stream imagery in browser compatible formats. However, these formats typically imply RGB JPEG or PNGs and services often require dedicated server-side resources. Not only do COGs neatly align with the shift to serverless solutions with minimal data duplication, frameworks emerge offering truly serverless web-apps – https://geotiffjs.github.io/.
Queryable Metadata
One might ask, there may now be millions of datasets becoming easily accessible, “streamable” even, via http protocols, but how might I discover what’s available and incorporate them within my own use case?
This is where the SpatioTemporal Asset Catalog (STAC) specification3, comes in. If COGs open the door for efficient access to image datasets, STAC helps users find the door in the first place. It’s a consistent, flexible and open specification within a disparate and convoluted landscape of geospatial metadata formats.
If image providers embracing the concept of ARD consider adding a STAC record to each cloud-optimised dataset, that dataset could become queryable directly from any browser or search engine. You can try this out by doing a google search for “planet disaster data hurricane harvey cc-by-sa-4.0 skysat geotiff” (Fig.1).
Together, COGs as a cloud optimised data format and STAC as a burgeoning metadata format offer a uniquely simple solution to that age-old geospatial challenge of improving and expanding data access. Such simplicity is key within a decentralised ecosystem of geospatial assets. The barrier of entry to non-traditional geospatial data providers and users is unequivocally lowered and the coupling of both technologies should lead to more user-centric innovation within a sector that will become, to (mis)quote Aristotle, ‘wholly greater than the sum of its parts’.
As COG is one option for addressing our first ARD component, STAC is just one option for the second. As we’re all aware, options for adopting a geospatial metadata format are numerous and often inflexible. The STAC specification differentiates itself in offering “a common language to describe a range of geospatial information, so it can more easily be indexed and discovered”. With the goal of “all providers of spatiotemporal assets (Imagery, SAR, Point Clouds, Data Cubes, Full Motion Video, etc) to expose their data as STACs, so that new code doesn’t need to be written whenever a new data set or API is released”.
Recall that the first massive-scale adoption of these first two components of ARD was Google Earth Engine (GEE) in 2010. Converting openly available geospatial image assets to a proprietary cloud-optimised format and hosting in Google cloud storage has enabled high-profile academic remote sensing research (think Global Forest Watch) and more than 700 academic papers4 (Fig.2).
We envision COGs and STAC as enablers for public and private organisations to realise these proven benefits alongside GEE and other geospatial platforms. This is supported by the recent ability for a user to input and export any COG from GEE. Nevertheless, there becomes the need to only make use of a platform where they offer an explicit, quantifiable value-add within a particular use case, whether commercial or non-profit, and shifting between alternative data sources or platforms will be trivial. Already we see that simply offering data access and use-case agnostic tools is insufficient to maintain a viable user-base.
The most significant public-sector commitment in this space is the much-anticipated publication by USGS of the Landsat Collection 25 archive onto AWS, in COG format and with STAC metadata (Fig.3). ESA is also exploring their adoption through private organisations funded by DIAS contracts and within its FedEO programme.
Scientific Quality and Provenance
The importance of public and private geospatial image providers addressing these first two components of ARD themselves cannot be understated. Making datasets more accessible by adopting these tools is only worthwhile when their content is of sound scientific quality and provenance. Another reason the Landsat Collection 2 is held in high regard is that it comprises fully-corrected surface reflectance products, as opposed to top-of-atmosphere images previously disseminated by USGS and at which the larger share of ESA’s early Sentinel-2 datasets continue to be provided. Increasingly users no longer need make per-scene corrections themselves to undertake robust multi-temporal analyses. This shift is further emphasised with Planet’s recent announcement of scientifically robust daily ‘fusion products’6.
Traditional private sector players, such as Maxar, Airbus and Telespazio, are involved in the development of COG and STAC to varying degrees. While the most significant drive comes from the now not-so-new space sector in the form of Planet, newer space companies such as CapellaSpace, ICEYE and Satellogic make clear assumptions that these technologies will evolve into standards they can readily adopt as constellations become operational. Whether this will take the form of closer collaboration with intermediaries such as SkyWatch than has, to-date been the case for traditional players, remains to be seen.
As Chris Holmes, organiser of the Cloud Native Geospatial Outreach Day7, poses the question: “what if geospatial tools and software were actually built from the ground up for the cloud?” We must envisage an ecosystem where it’s ever easier to develop innovative user-centric geospatial solutions that incorporate EO datasets just like any other web-hosted asset. Further challenges – and opportunities – ensue for new and existing public and private sector value propositions. Here in the UK these evolutions will be key to unlocking the estimated 1.2 Bn of value that EO has been estimated to offer to the public sector and maintaining industry growth in-line with the European 11.5% CAGR8.
5. https://www.usgs.gov/core-science-systems/nli/landsat/landsat-collection-2
6. https://www.planet.com/pulse/planet-announces-powerful-new-products-at-planet-explore-2020/
7. https://medium.com/radiant-earth-insights/cloud-native-geospatial-outreach-day-recap-9b873ce3788e