The regeneration of forest saplings is pivotal in maintaining biodiversity and ecosystem productivity, yet traditional 2-dimensional remote sensing struggles to accurately capture the complex, understory sapling dynamics. To address this, researchers are exploring the potential of Aerial Laser Scanning (ALS) to provide detailed 3-dimensional insights
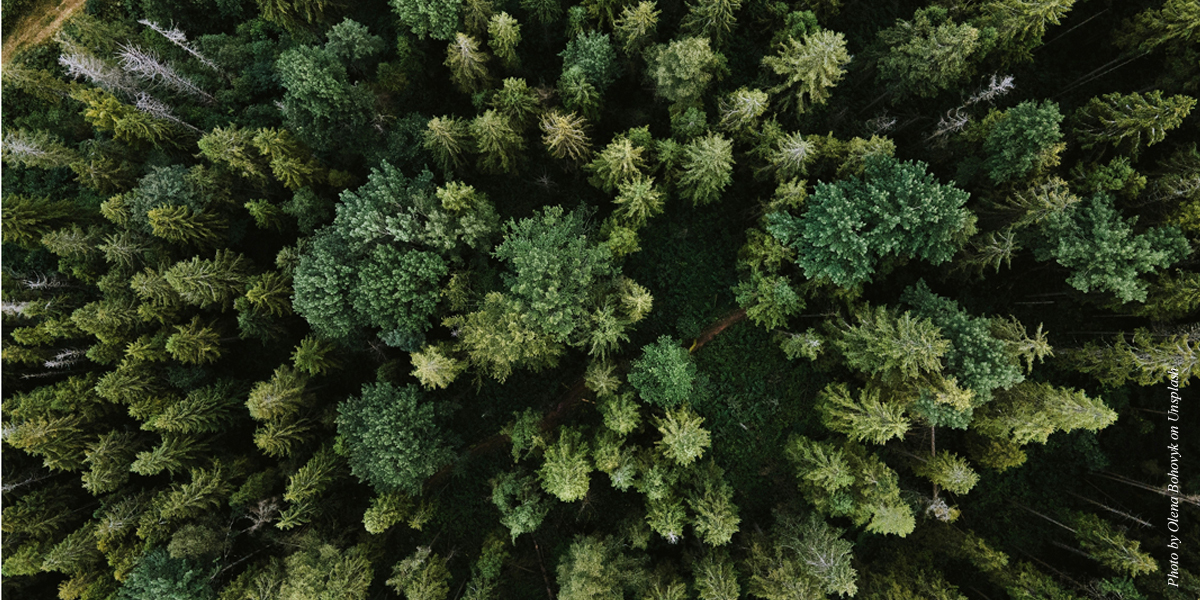
Despite progress in using ALS data to estimate tree metrics, the ability to accurately identify and quantify the phenotypic parameters of understory saplings remains a challenge. The current research gap lies in refining and applying algorithms that can effectively distinguish understory saplings within ALS datasets, a critical step towards enhancing forest management and understanding sapling regeneration processes.
Plant Phenomics published research titled "Identifying Regenerated Saplings by Stratifying Forest Overstory Using Airborne LiDAR Data."
In this study, researchers at China's NanJing Agricultural University developed a comprehensive methodology (pictured below) to extract phenotypic parameters of understory regeneration saplings using advanced high-density airborne LiDAR data. Initially, they fused data from multiple flights to generate a dense laser point cloud, which was then processed using a Nyström-based spectral clustering algorithm for segmenting upper mature trees.
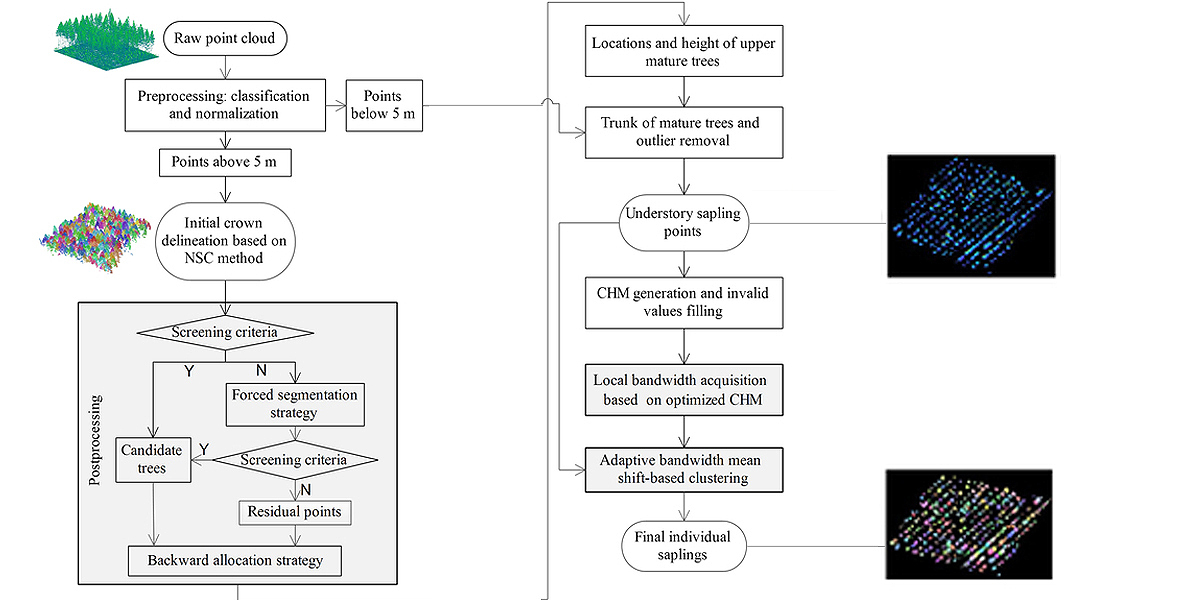
To address the common issues of oversegmentation and undersegmentation, a novel postprocessing method was introduced, prominently enhancing the positional accuracy of mature trees.
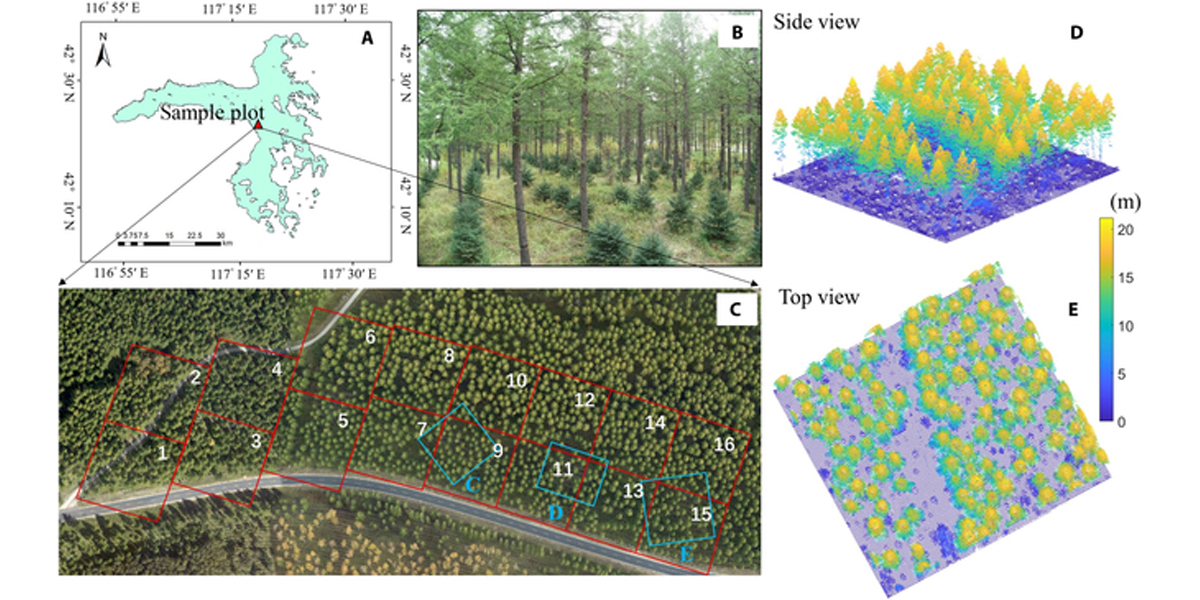
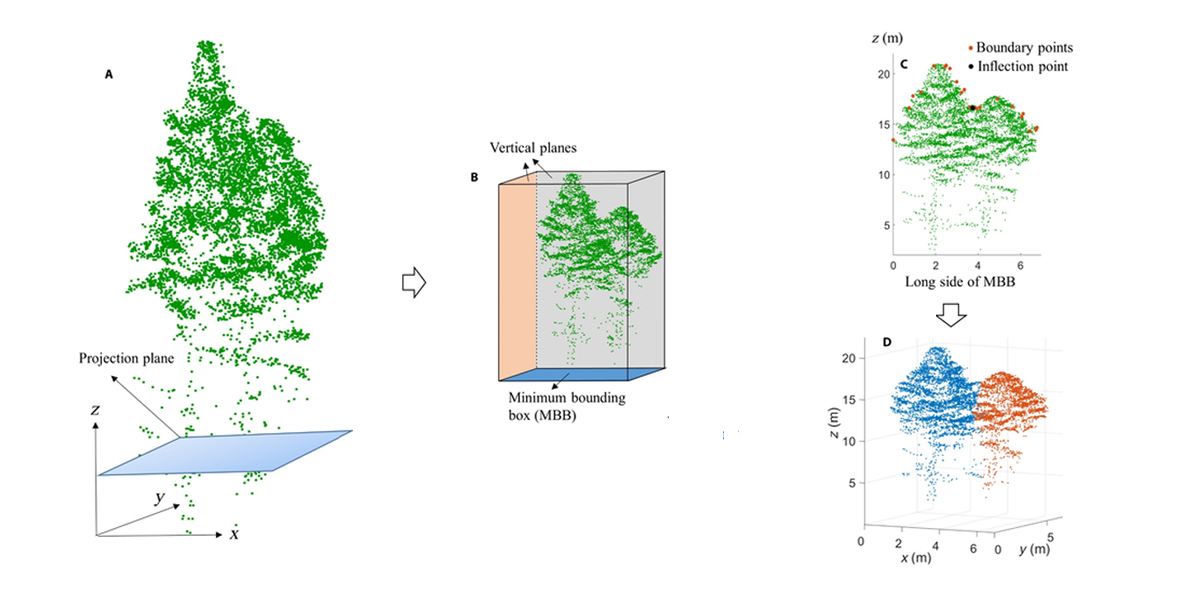
The results were impressive, showing a notable improvement in the detection and matching rates of tree segmentation after implementing the postprocessing steps; the detection rate for the Nyström-based Spectral Clustering Postprocessing (NSCP) method reached 110.21%, with a matching rate of 96.69%.
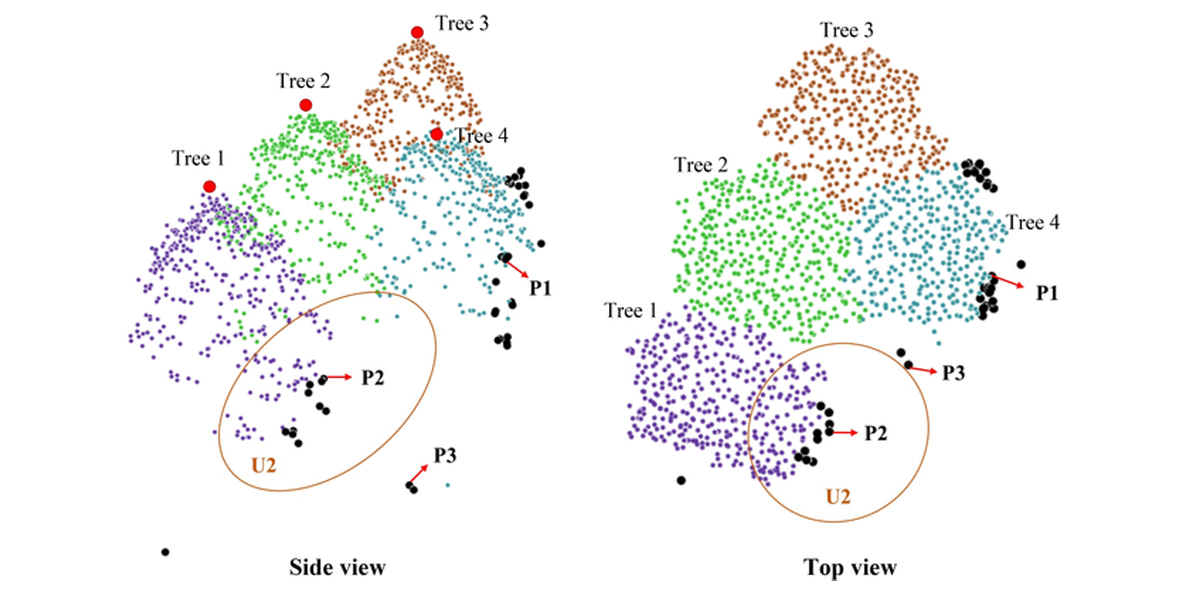
This optimization reduced trunk position errors, indirectly benefiting the accuracy of understory sapling detection. Utilizing the local adaptive mean shift algorithm, saplings under mature trees were successfully detected, achieving a matching rate of approximately 83% with an extraction rate between 102% and 105% when the kernel bandwidth parameter was optimally set.
Further validation using multisource reference data confirmed the method's efficacy. Comparisons between airborne LiDAR (ALS) and terrestrial laser scanning (TLS) data revealed a strong correlation (R2 = 0.79) for tree height, demonstrating a notable improvement in RMS error after accounting for the ALS's inability to detect terminal leader shoots.
This adjustment led to a more accurate representation of sapling heights, with an overall R2 of 0.71 and RMSE of 0.26 m when comparing ALS-extracted sapling heights against field measurements. Additionally, sapling crown widths estimated from ALS data, when matched against TLS measurements, yielded an acceptable R2 of 0.64 and RMSE of 0.24 m, despite the challenges posed by upper canopy obstruction.
In conclusion, the study not only demonstrated a successful application of high-density ALS data for understory sapling characterization but also highlighted the potential of this technology to enhance forest management and sapling growth studies. The proposed method distinguishes itself by accurately segmenting and measuring understory saplings offering a significant step forward in the use of remote sensing technologies for detailed forest inventory and analysis.
More information: Liming Du et al, Identifying Regenerated Saplings by Stratifying Forest Overstory Using Airborne LiDAR Data, Plant Phenomics (2024). DOI: 10.34133/plantphenomics.0145
Provided by NanJing Agricultural University
Subscribe to our newsletter
Stay updated on the latest technology, innovation product arrivals and exciting offers to your inbox.
Newsletter