Researchers from the Leibniz Centre for Agricultural Landscape Research (ZALF) in Müncheberg, Germany, together with international partners, have developed a new framework that significantly improves landslide prediction using Machine Learning methods
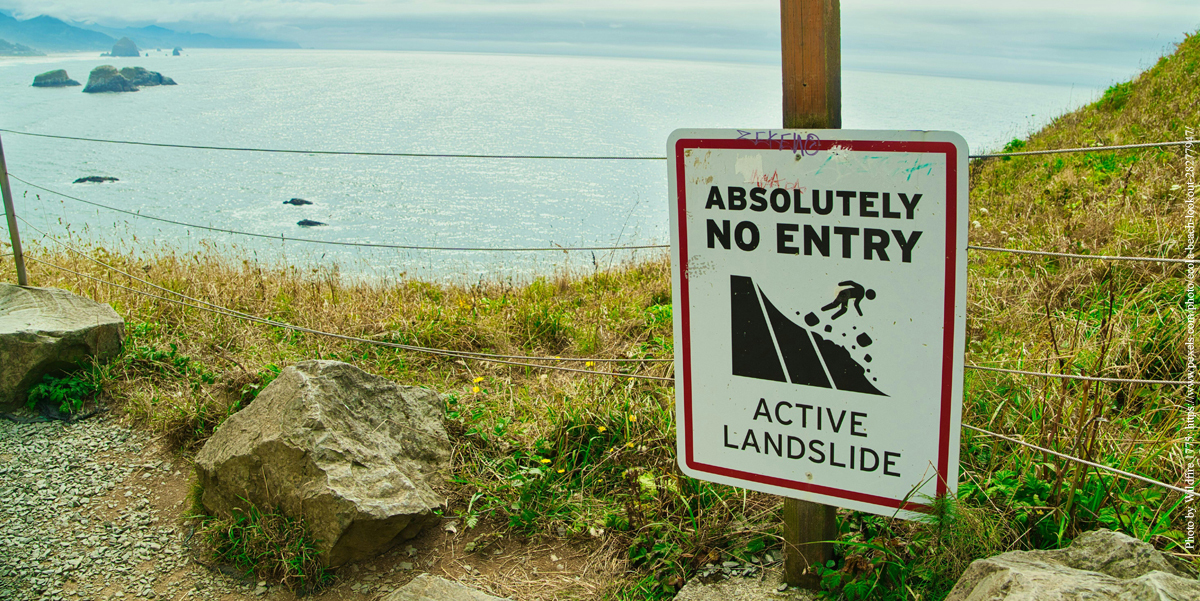
The model can analyze data and create precise maps highlighting landslide-prone zones. It achieves an impressive 95.6% accuracy in predicting landslide risks. The results are published in the journal Scientific Reports.
How does the model work?
To better predict landslide risks, the model uses a combination of six different machine learning methods.
A simple way to understand this is to compare it to a weather forecast. If you want to predict tomorrow's weather, you analyze past weather patterns and look for indicators—like dark clouds and strong winds often signaling rain.
This model does something similar—but for landslides and on a much larger scale. It processes vast amounts of environmental data, such as:
• Rainfall levels: Where does it rain the most?
• Soil composition: Is the soil stable or loose?
• Landscape slope: How steep is the terrain?
• Vegetation cover: Are there trees stabilizing the ground, or has deforestation occurred?
• Human activities: Are there roads or buildings that make the area unstable?
The model compares this information with past landslide events, recognizing patterns that indicate high-risk areas.
A meta-classifier then leverages the strengths of multiple AI models by combining their most accurate predictions to enhance overall performance. It works in three key steps:
1. Training base models—Several machine learning models (e.g., logistic regression, support vector machine, random forest, extremely randomized trees, gradient boosting, and extreme gradient boosting) are trained independently on the dataset.
2. Generating meta-features—The predictions from these base models are used as new input features.
3. Training the meta classifier—A final predictor (e.g., logistic regression) is trained on these aggregated predictions to make the final decision.
"With our new prediction models, we can identify landslide-prone areas much more accurately than before," explains Krishnagopal Halder and Dr. Amit Kumar Srivastava from ZALF. "This is an important step towards better protecting people and enabling sustainable land use."
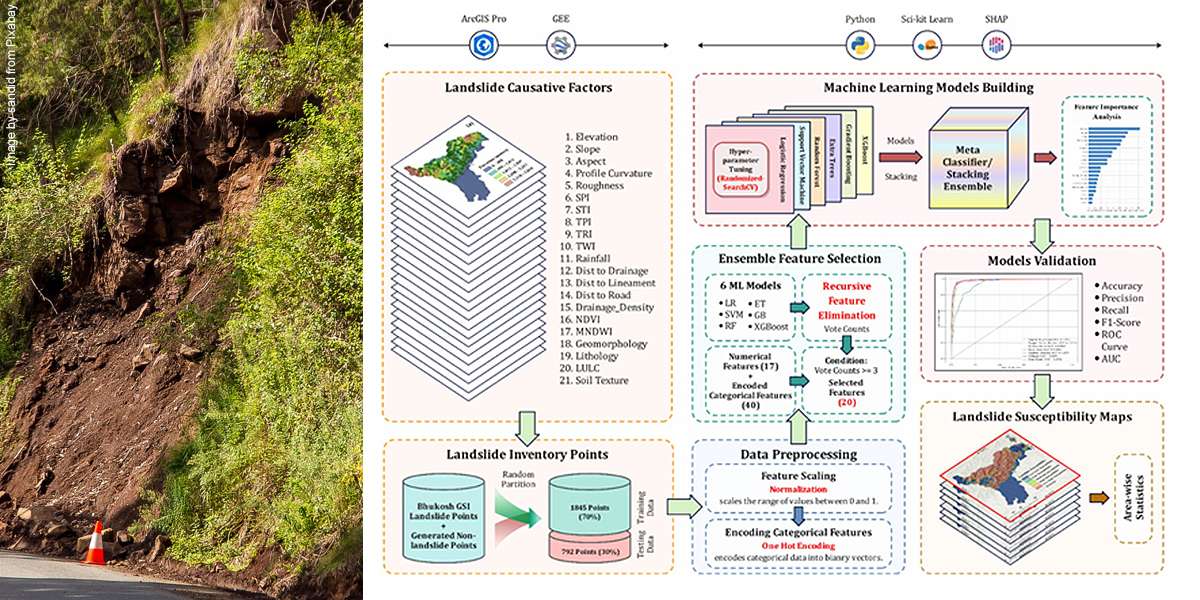
Why is this model so important?
Landslides often occur suddenly and can cause significant damage. Traditional risk assessment methods are often inaccurate or take a long time to complete. The new model can analyze vast amounts of data quickly and achieves an impressive 95.6% accuracy in predicting landslide risks.
The research team tested the model in the Sub-Himalayan region of West Bengal, India—an area heavily affected by landslides. The analysis revealed that high-risk zones are primarily located in areas with heavy rainfall, unstable geological structures, and intense land use, such as deforestation and urbanization.
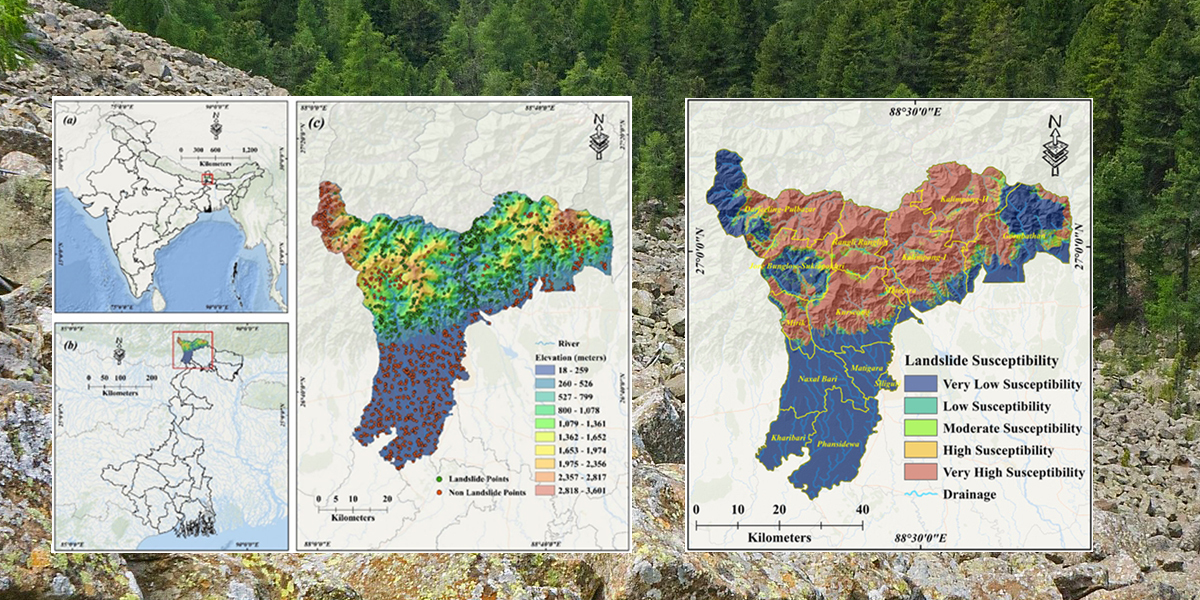
By using this new technology, authorities and disaster management organizations can take early action to secure vulnerable areas and issue warnings in advance.
The method can be applied not only to landslides but also to predicting other natural hazards such as floods or land subsidence. In the future, the model could be further refined and adapted for global use.
Project partners:
Leibniz Centre for Agricultural Landscape Research (ZALF), Germany
University of Bonn, Germany
Coastal Environmental Studies Research Centre, India
Columbia University, USA
Chhatrapati Shahu Ji Maharaj University, India
The University of Burdwan, India
Bhatter College, India
Funding: This research was supported by the German Research Foundation (DFG) and the BMBF project BonaRes.
More information: Krishnagopal Halder et al, Improving landslide susceptibility prediction through ensemble recursive feature elimination and meta-learning framework, Scientific Reports (2025). DOI: 10.1038/s41598-025-87587-3
Journal information: Scientific Reports
Story Source: Leibniz-Zentrum für Agrarlandschaftsforschung (ZALF) e.V.
Subscribe to our newsletter
Stay updated on the latest technology, innovation product arrivals and exciting offers to your inbox.
Newsletter